Energy System Modelling for UK Industrial Decarbonisation
To deliver the low-carbon UK energy network, we need to move beyond isolated static plans and utilise new innovative technologies.
Investment into the UK energy network will unlock a low-carbon future. To deliver that requires us to move beyond isolated static plans, utilising new innovative technologies.
- A gap exists between the strategic national scenario modelling (e.g. FES) and the regional modelling required to inform and drive local energy system infrastructure development.
- Energy system models need to enable collaboration between key infrastructure providers to support intelligent, joined-up planning and development activity at a regional level.
- Models must include more accurate future demand data by integrating economic models.
- AI can help build more accurate future demand profiles at a regional level.
- Models need to be kept dynamic and live to provide the best information through digital interfaces, not static reports.
- Collaboration across the energy vectors is key – local clusters can help facilitate this.
Future Energy Strategy
Investment in the energy network is essential for long-term, low-carbon, low-cost energy. However, there are short-term challenges for investment and planning across the wider energy system development, which need immediate resolution to deliver effective strategies.
The development of the UK’s energy network can unlock long-term sustainable growth, facilitating the plentiful supply of low-cost, low-emission energy. There is further opportunity to facilitate the expansion of decentralised renewable energy, helping increase energy security, and reduce CO2 emissions, the overall cost of energy and a just transition.
A collaborative and dynamic approach at a regional level to energy system modelling can accelerate the transition to a resilient, low-carbon energy network.
System Complexity
The UK energy landscape is a complex mix of stakeholders - infrastructure developers, network operators, energy regulators, energy consumers, technology innovators, and central and local government. This creates a challenging planning environment where different stakeholder needs and considerations are potentially overlooked.
For example, distribution network operators (DNOs) conduct strategic planning through DFES. This is a powerful model base that enables integration with other energy vector initiatives (e.g. gas or hydrogen) and CO2 sequestration projects at a regional level.
Consider the planning challenge of integrating data and feedback from regional economic growth strategies - their local variance, data quality and availability - to ensure DNOs can plan and enable the energy provision to facilitate this growth.
Building Scenarios
The National Grid Future Energy Scenarios (FES) is an excellent resource to understand what different energy systems and the associated impacts could look like under alternative conditions. However, it isn’t designed to support regional planning and investment decisions on new technology and infrastructure deployment.
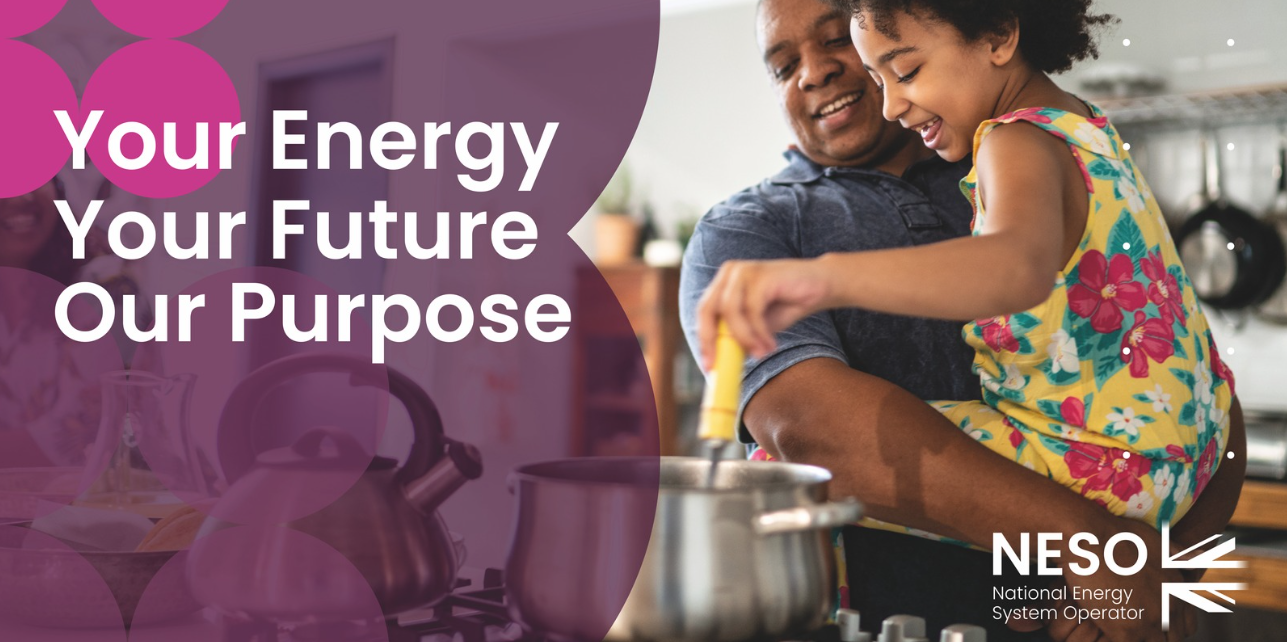
Add to the scenario the unknown pace of development of alternative low-carbon energy (e.g. green hydrogen) and CO2 sequestration technology - the additional variables, complexity and uncertainty to network planning is exponential.
A key integration gap exists that needs bridging - between the strategic national scenario modelling (e.g. FES) and the regional modelling required to inform and drive local infrastructure development.
Planning Challenges
Operators and developers typically plan in isolation (Figure 1) when confronted with such complexity and uncertainty. This compounds the challenge of transitioning to a more connected, smarter, low-carbon energy system.
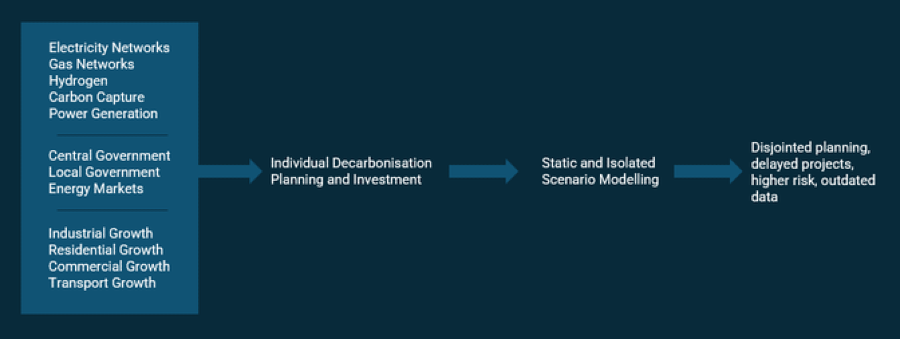
The establishment of the Future Systems Operator NESO, will help to address this challenge, as will the development of a Strategic Spatial Energy Plan (ref ). Moving through the system hierarchy, to reach and support the foundational organisations (DNOs, developers etc.) further strategic support and action are needed to enable proactive investment decisions.
Regional Strategies
A solution to this is the development of regional clusters that facilitate organisational cross-collaboration. Ada Mode is developing a comprehensive dynamic energy system model with the Solent Cluster to facilitate the region’s Local Industrial Decarbonisation Plan.
A dynamic model that captures the complexity of a regional energy system is essential to underpin effective planning activity (Figure 2).
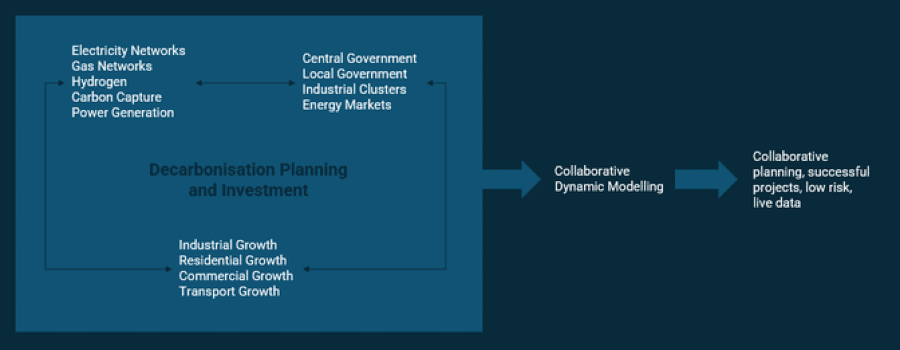
Dynamic Modelling
Key requirements of a dynamic model to enable this level of complexity;
Regional economic growth data can be challenging to acquire and inconsistent in format - in such cases, previous growth and demand data can be used to create a forecast based on business as usual.
Energy demand predictions with traditional models are often too simplistic – driven by limited data analysis and trends. By integrating novel machine learning approaches in load forecasting, predictive models take into account more dynamic variables. This creates more effective and robust models, with flexible dynamic scenario capabilities.
“Potential Futures” analysis is more accurate when utilising the full capabilities of AI modelling. Standard historical data models will show increased demand during winter. AI modelling allows for analysis of how demand affects system assets, such as integrated solar PV, alongside increased heating demand.
Integrating linear optimisation methods can help reach a better predictive model as potential future pathways are analysed - key actions using linear optimisation are performed. This allows for analysis using different scenarios, e.g. what is possible via maximum capital investment vs a more balanced cost-efficient option?
Future Outcomes
The Solent region dynamic model supports decision-making for all stakeholders and organisations that can influence the overall energy system. It will help developers of new energy generation facilities or infrastructure for hydrogen or power plants understand demand while also providing network operators with capacity over time with the integration of regional economic growth.
It will help large, hard-to-abate sites consider the techno-economic use case of carbon capture and present a view of how the power grid will grow and change over time. It will also help individual industrial sites' future planning with insights into the expansion and availability of regional low-carbon energy.
Additionally, as new technology innovation in energy seeks access to the grid and future commercial markets, dynamic modelling can deliver insights for pilot, strategic and project planning purposes, by regional need and opportunity.
This holistic approach to cluster or regional dynamic modelling can enable stakeholders in other regions to accelerate the path to net zero through smarter planning and investment.
About the Author
Jack Lewis
A chartered mechanical engineer with a history of power station management and leadership of complex engineering projects in nuclear and renewable power generation. A creative thinker with a background in engineering design, product development and systems design.
Co-Founder of Ada Mode - an AI company with a mission to accelerate the journey to net-zero through improving the performance of complex industrial facilities in renewable energy, civil nuclear power, water and manufacturing
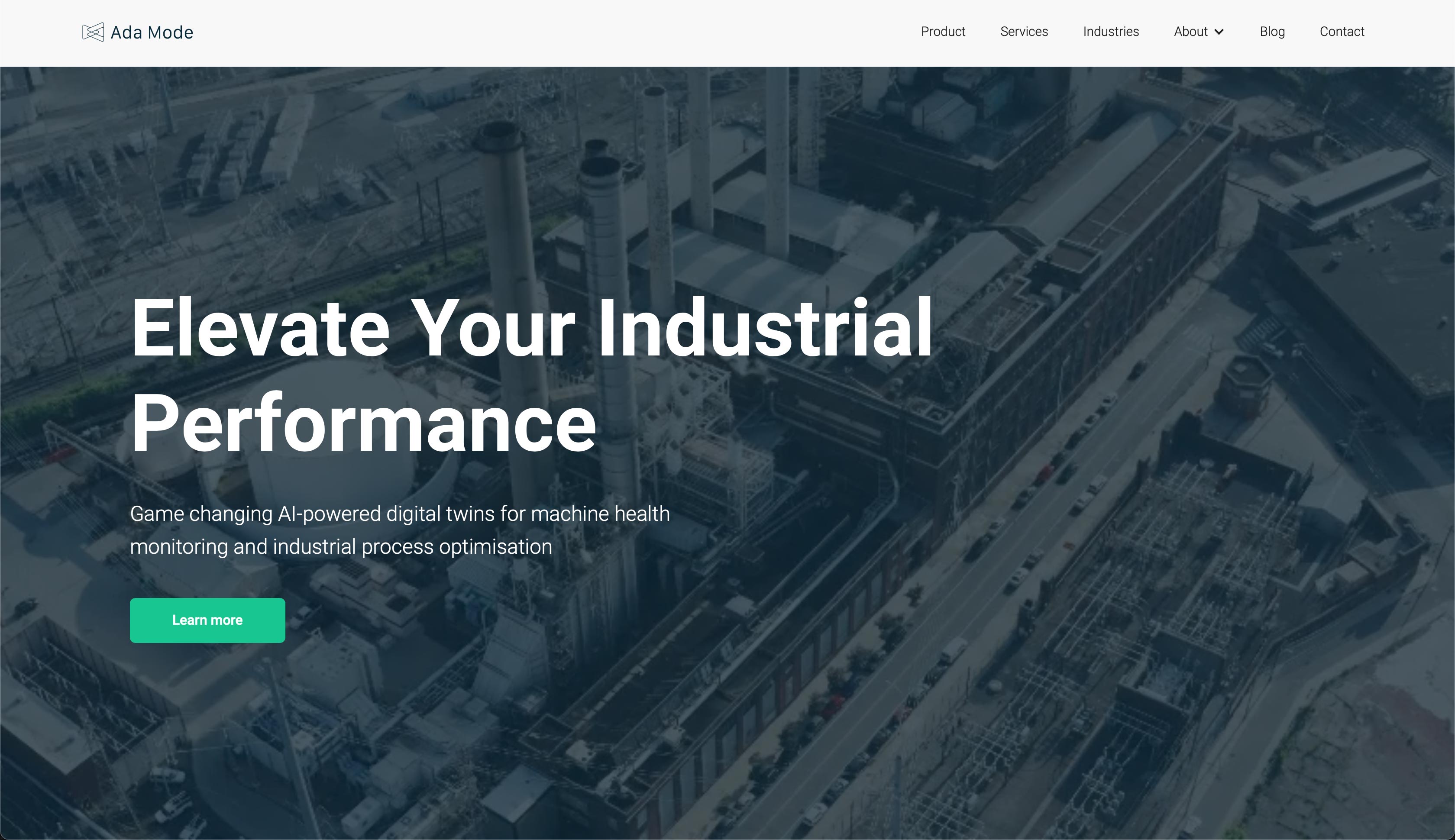
Co-Founder of Windscope - a SaaS platform with a mission to reduce the cost and improve the safety of wind turbine O&M activity through next-generation system health monitoring.
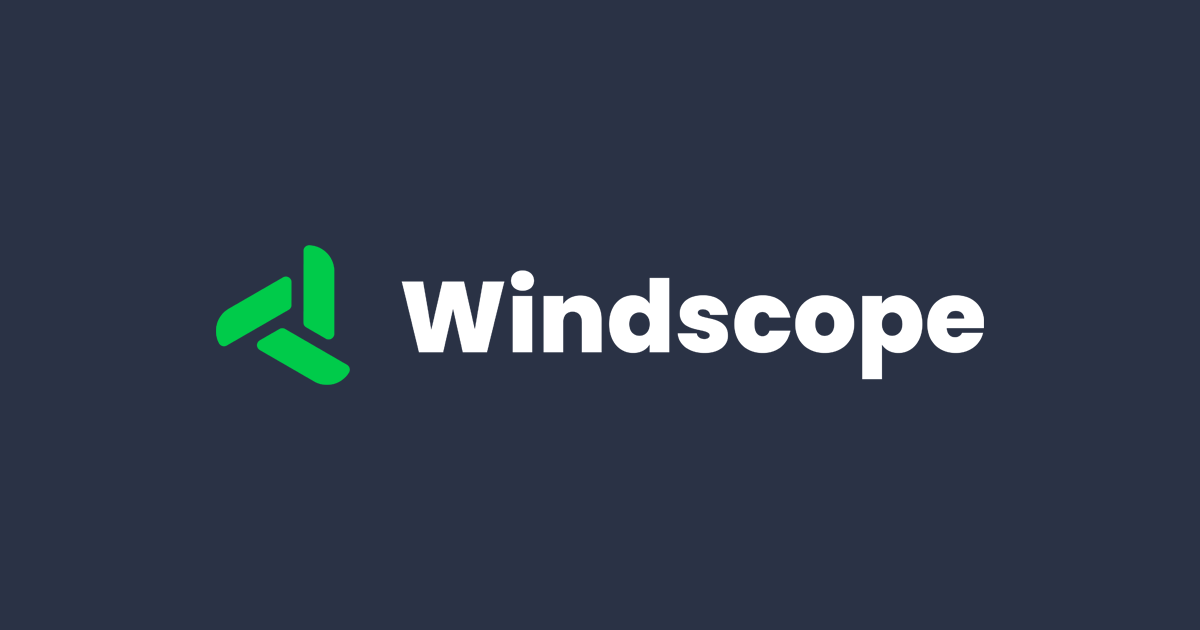